Big Challenges, Big Opportunities: Big Data
by Jeff Tuzik
Raw data by itself doesn’t always help much. In fact, it can be a burden. Extracting actionable information from raw data is an issue that the railroad industry has grappled with for years. (See WRI 2008: Data to Information) And as measurement and monitoring technologies have advanced, become cheaper and more ubiquitous, “data-to-information” has morphed into a broader discussion about how to manage Big Data. Class 1 railroads, including CSX Transportation, Union Pacific and Norfolk Southern, have formed an informal working group specifically to address Big Data. But they’re not alone in addressing the matter.
The University of Delaware recognized the issue and in December 2014, held a conference: Track Maintenance and Planning in the Era of Big Data. The conference, hosted by Allan Zarembski, the university’s Director of Railroad Engineering and Safety, and Nii Attoh-Okine, Professor of Civil and Environmental Engineering, brought together industry professionals, suppliers, and academics to discuss some of the challenges and opportunities that Big Data presents.
“Twenty five years ago, nobody had Big Data. Nobody had enough data,” said Joseph Palese, Senior Director of Inspection at Harsco Rail. As the amount of easily accessible data has increased over the years, there have been some great success stories, he said. One example is the evolution of rail grinding, a technique that draws on disparate data sets (rail wear and wheel wear, L/V, profile and geometry measurements) and combines them into a system of wheel/rail interface optimization and wear reduction.
“You need to be able to connect disparate pieces of data in order to see the big picture,” Allan Zarembski said. And that’s one of the challenges of Big Data; it’s hard to see the big picture when the details are overwhelming.
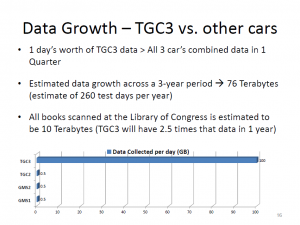
CSX Transportation’s TGC3 generates a tremendous amount of data every day due in part to its imaging and machine vision technologies. (Courtesy of CSX Transportation)
“Too much data can make it hard to make the right decisions,” said Matt Dick, Staff Technical Officer at ENSCO. Due to the tremendous amount of data collected by monitoring and measurement systems, it would be impossible to act on all of it even if the actionable information were clear. The trouble is, it’s rarely clear; the sheer amount of raw data can obscure the right decisions and actions.
“Mass data can be an asset, but it has to be turned into information and the information has to be turned into decisions,” said Dan Magnus, Vice President of KLD Labs. Part of the way forward in dealing with Big Data has to do with removing the “human bottleneck” from the equation. With giga- and tera- and soon petabytes of data flowing in and collecting on servers, the notion of people sifting through the data to generate actionable information is increasingly unfeasible, he said. Greg Mellish, Assistant Chief Engineer of Inspection Processes at CSX Transportation, acknowledged that fact noting that CSX’s latest Track Geometry Car, which incorporates machine vision, thermal imaging and video in addition to standard measuring devices, generates 100gb of data in a single day of testing.
John Kainer, Manager of Aurora Development at Georgetown Rail Equipment Company, echoed that assessment saying, “With humans doing all the data processing and management, the result is a non-scalable system.”
One of the problems with the “non-scalable” system currently in place is that potentially useful information may be sitting undiscovered in data that has already been collected. Matt Dick referenced a recent ENSCO program that discovered a pattern of clusters of low-level exceptions that preceded particular derailments. By applying an algorithm that searched for similar exception clusters to existing data troves, the program predicted the location of another derailment before it occurred. It’s a stark example of the information that can be gleaned from previously-collected data with sophisticated data management.
Clearly decisions made without that type of “hidden” information may not be an optimal use of resources. “When we send people out to do maintenance, we have to be sending them to the worst, highest priority places,” said Dwight Clark, UP’s Assistant Chief Engineer.
Another unfortunate aspect of the “non-scalable” system is that there is a disincentive to collect more data than is currently manageable or actionable, Matt Dick said. It’s a problem that highlights the importance of having automated systems to comb through data. “There’s a need to automate the decision making process, not just the data collection and processing,” he said. Singling out the highest-priority areas, for maintenance for example, means taking into account the totality of data collected from disparate sources. In other words, the notion of “too much data” could, and should, be made moot through automated processing and decision making.
Bringing automation and machine intelligence into data processing and management has the added benefit of taking subjectivity out of the equation. “It’s easy to spend a lot of money in the wrong place just because some one’s a better arguer than another,” UP’s Dwight Clark said.
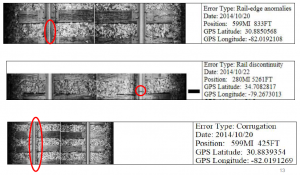
Automating data processing and analysis, as in the system pictured, is a necessary step in removing the “human bottle-neck” from the equation. (Courtesy of CSX Transportation)
Tony Cabrera, Director of Track Engineering at NYCT, noted that the subjectivity and “human bottleneck” of visual tie inspections prompted the NYCT to employ a series of machine vision units on one of their inspection vehicles. However, there are not yet automated systems in place to process the collected data, he said. The problem now? Too much data.
It may be that artificial and computational intelligences are the “holy grail” of data and decision management. But until those technologies mature, increasingly sophisticated algorithms and methodologies are helping to make sense of data-overload in the railroad industry. Professor Nii Attoh-Okine presented, for example, algorithmic methods for reducing noise and highlighting edge-detection in rail flaw images. The resulting cleaned-up images can make flaws easier to detect for both human and machine processing. “You have to squeeze the data until they tell you the truth,” he said.
We look forward to future iterations of this conference at the University of Delaware. The conversation about the big challenges and big opportunities associated with Big Data will also be taken up at WRI 2015, with Dr. Zarembski leading a panel that will include railroaders, researchers and suppliers to continue to move the discussion forward.
Jeff Tuzik is Managing Editor of Interface Journal