Moving to Performance-Based Automated Track Inspection: The Benefits and Hurdles Ahead
By David C. Lester
The mileage of individual Class 1 railroad networks presents a challenge to inspectors and engineers to inspect frequently enough to keep the track in good shape and minimize the frequency of track-related derailments. Traditional inspections done by railbound geometry cars and flexible hi-rail trucks, with the results interpreted by long-experienced track inspectors are now difficult to do at the necessary intervals because of the talent drain resulting from retirements and turnover of experienced track inspectors, and the increasing difficulty of obtaining track time. As a result, scarce capital planning dollars must be focused on the correct places.
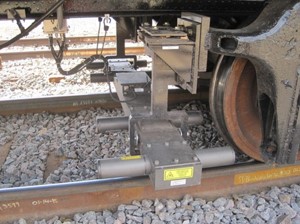
Today, in addition to traditional track inspection methods, Class 1s are supplementing their fleets with unmanned geometry cars and vehicle / track interaction equipment mounted on locomotives. Moreover, the software to support these systems is complex. Physical track inspection generates a great deal of data, and this data must be evaluated for integrity, and adequate storage must be available. The challenge is to use data analytics to obtain information from all of this data that will add more precision to the capital planning process.
BNSF, for example, currently uses three crewed geometry cars, three uncrewed cars, four hi-rail geometry trucks, and vehicle / track interaction (VTI) equipment mounted on 61 locomotives. The railroad also brings in a few outside contractors to perform supplemental inspection work. The crewed and uncrewed railbound geometry cars are equipped with precisely the same equipment, which includes truck-mounted geometry and rail profile beams, laser illumination with multiple cameras, and multiple display screens for field evaluation as information is collected and analyzed.
The company also makes extensive use of visual evaluation systems. These include monochrome line scan cameras with lighting systems to perform joint bar and other track component inspections, and analysis of manual and machine vision to evaluate the condition of the track.
One of the key goals for using automated vision systems is to transform manual inspection into condition evaluation. The automated system must collect, analyze, and determine a plan for remediation. The system must detect the track feature (e.g., joint bar, tie, etc.), and determine if there is an exception based on predetermined rules.
In addition to systems that look closely at track, BNSF has had an Unmanned Aerial System (UAS, or drone) program in place for over six years. The drones are used primarily for service interruption support, slope and material buildup monitoring, distance, volume and area assessments, and water runoff direction and flow rate determination. BNSF has trained drone pilots throughout their territory who are available 24/7/365; if an event that calls for quick inspection occurs, it takes about 45 minutes from the time a call for a drone is made until the pilot and drone are in place and ready to fly. In addition to providing a first look at the trouble, the drones provide first responders and remediation support and provide necessary information in case other aircraft or the corporate jet fleet needs to be activated. And, the UAS program eliminates a lot of personnel travel.
The unmanned aerial system is also used for bridge inspection on BNSF. With automation software, the drones can identify missing rivets, cracks in concrete, rust bleeding, excessive corrosion, and pitting, along with cracks in steel. The software can also provide automatic pixel-level change detection that identifies any changes in a rust bleeding pattern, along with any changes in or deformations of structural components.
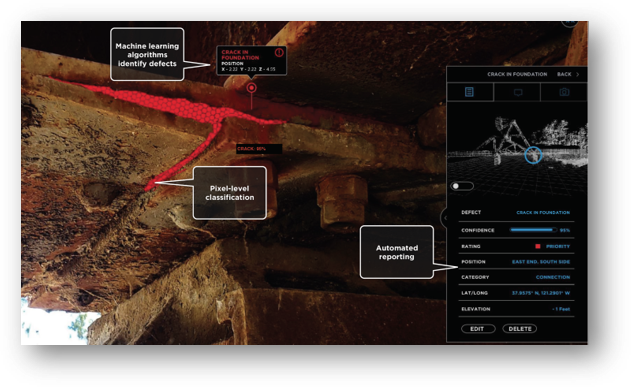
Water flow monitoring around bridges is another benefit of the UAS program. As time passes, sediment will build up around bridges. Drone images can identify sediment buildup that can interfere with water flow under and around the bridge, or debris accumulation after a storm.
BNSF also uses the UAS for slope monitoring. One example is the proximity of their track to a Quarry in Birmingham, Ala. The drone provides monitoring of the distance from the track to the quarry ledge to enable engineers to know when to take action to keep the quarry a safe distance from the track.
A relatively new technology now used by BNSF is called Light Detection and Ranging (Lidar). The system physically measures and classifies the track structure and identifies objects. Lidar uses lasers to collect information and measurements that are then used to create 3D models for visual presentation. Key applications of Lidar are PTC asset mapping, track asset management, high-wide clearance issues, drainage profiles, turnout condition assessment, and ballast profiles.
Another item in the BNSF toolbox, as well as in the toolkits of most Class 1 railroads, is Ground Penetrating Radar. The antenna frequency determines the depth of evaluation, and this tool is excellent for evaluating tie condition, as well as the condition of ballast and even sub-ballast.
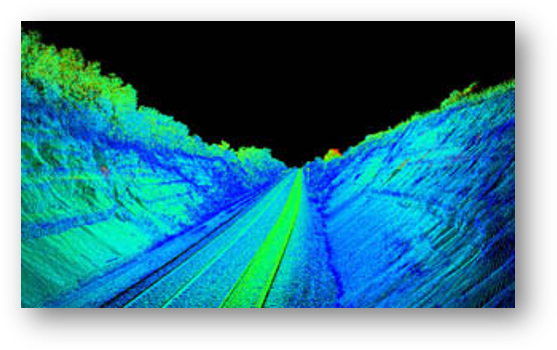
BNSF says that the key areas for automation development today include selecting the correct sensor for a given application, greater use of edge computing, (both hardware and software) which keeps the computer processing power closer to the data collection device (as opposed to a server thousands of miles away) to enable faster data processing and reduced latency. Also, the cloud needs to be leveraged more, particularly because of the massive amount of computing horsepower that’s required to process the massive amounts of data being collected, said Mike Mischke, recently retired BNSF senior consulting systems engineer. This lowers the cost of processing by not having to invest in powerful computing hardware, which will likely become inadequate in a short period due to the increasing amount of data collected for processing. Finally, systems to perform analytics on data from machine vision are needed to process and analyze the massive amount of data acquired during inspection runs, he said.
When a geometry train or other roadway inspection vehicle makes a run, various types of data need to be collected at the same time and have the same timestamp.
“We’re moving towards comprehensive evaluation platforms, vehicles that collect many different things instead of just one or two different things,” Mischke said. “What this does for us is basically aligns the data very well and gives it the same time stamp, which makes it easy to evaluate.”
For example, track video; rail, tie, ballast, and ground-penetrating radar imagery; lidar data; geometry and rail profile; and machine vision identification of assets can all be collected concurrently for a comprehensive evaluation.
Planners are looking forward to the arrival of new technologies in the coming years, such as new types of lidar and cameras that have been developed by the automotive industry for driverless cars. Locomotives will continue to play a more significant role in track evaluation through the addition of full geometry and machine vision equipment. New sensors, along with multiple types of radar and cameras that capture information beyond visible light, are under development, and spaceborne imagery and sensor data will be available for rail maintenance planning in the not-too-distant future.
The value of data is diminished if it’s siloed. Hence, data alignment is becoming more critical because aligning the data from all data sources provides better insight into track conditions. Rail profiles, video, imagery, point clouds, linear data, along with maps and schematics, when evaluated together, provide a near-complete picture of track condition that one cannot obtain with just one or two data sources.
Some of the challenges of evaluating track conditions and planning maintenance expenditures using data analytics are:
- What problem is one trying to solve?
- What data or technology should be used to provide insight into the problem?
- When you can’t obtain the data you’d like, can you solve a problem with the data you have?
- Data movement and management
- What needs to be kept for future analysis?
- Resolution of video and imagery; driven by analysis and visualization.
- Aggregation and consolidation of data for extracting additional value.
- Data is siloed in multiple repositories in many places.
- Change management to educate affected users who can best utilize the data.
About two years ago, BNSF rolled out what they call a Comprehensive Surface Plan (CSP), which has been a game-changer for them. The plan took about a year to put together, and it required input from operations research resources, as well as experienced roadmasters, and allows them to take a much more comprehensive approach to track maintenance, said Frank Moffitt BNSF’s recently retired General Director of Maintenance Planning. With limited track maintenance windows, the CSP puts a premium on precise execution. For example, if a track gang is working on a defect or exception, it’s essential to work the entire track segment rather than addressing only the exceptions because there could be other issues that haven’t popped up yet. MOW departments don’t want to have a track gang 20 miles down the road taking care of exceptions only, then have a slow order pop up behind them, Moffitt said.
Even though the CSP was rolled out two years ago, and the team has initiated an intensive effort to educate the track forces on the model, the railroad has reached about a 60 percent usage level with the model. They classify their track issues similar to the FRA in that red tags are limited to track defects, orange tags are a much higher-level exception that is not a defect, and yellow tags are maintenance exceptions. BNSF developed orange tags a couple of years ago, and they help focus inspections and prioritize surfacing behind a geometry car. Orange tags represent track conditions that have higher priority than yellow tags but do not reach red tag limits.
Predictive analytics are utilized with yellow tags to predict whether or not the yellow tag will turn red within 30 days. The surface parameters include cross level, surface, and alignment; warp is coming soon. Gage, cant, and rail wear have orange tag limits established halfway between red and yellow.
BNSF also uses a regression model to predict rail wear. The challenges of predicting rail wear include track alignment, rail weight discrepancies, and train model data sets. Variables the model uses are rail wear as measured by the geometry car, tonnage (MGT), degree of curvature, and since the model is not linear, the model accommodates the acceleration of wear. BNSF is developing the model to identify rail wear discrepancy fixes, and the data will be presented on engineering track charts and Tableau reports.
The railroad replaced about three million ties in 2018. Data analytics is supporting the decision around whether a tie should be replaced and helps those in the field mark the ones that do. Tie data is collected, analyzed, massaged, and loaded into a database. The tie condition is expressed as good, marginal, or bad. The data is then downloaded to a handheld device for marking the ties, and this information is conveyed to tie gangs.
Turnout reliability is another condition for which analysis is supported by analytics. The model compiles all different metrics that speak to the condition of a turnout and has a scoring metric for different defects and other data points.
Assessment using automated evaluation reduces the amount of field evaluation with virtual field inspection, defines remedial action based on condition, and uses environmental and tonnage factors to predict condition evolution. The goals of the CSP model are to improve the capital planning and maintenance-of-way processes, defining what needs to be corrected, and providing clear and understandable communication to the field.

This article is based on a presentation made at the WRI 2019 Heavy Haul Conference.
David C. Lester is Managing Editor of Railway Track and Structures.